
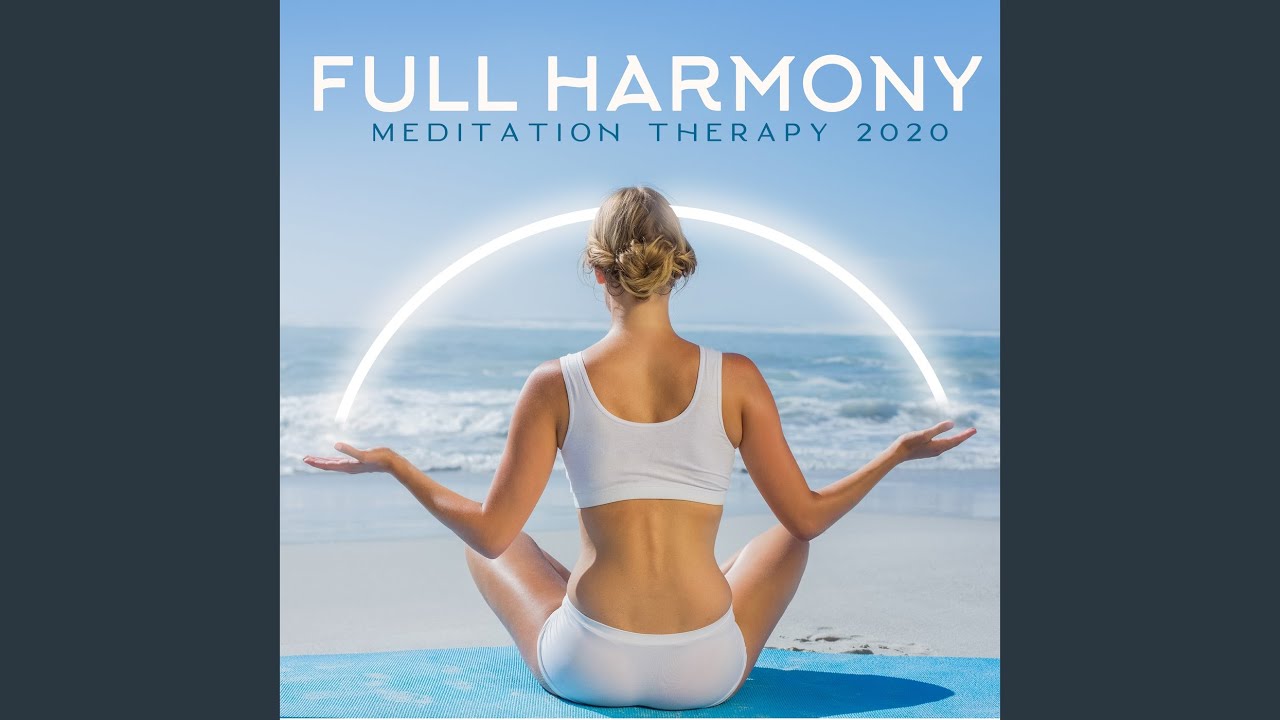
The RTX 2080 Ti, on the other hand, is like a Porsche 911. It's one of the fastest street legal cars in the world, ridiculously expensive, and, if you have to ask how much the insurance and maintenance is, you can't afford it. 2080 Ti is a Porsche 911, the V100 is a Bugatti Veyron Why would anybody buy the V100? It comes down to marketing. Most use something like ResNet, VGG, Inception, SSD, or Yolo. Fewer than 5% of our customers are using custom models.
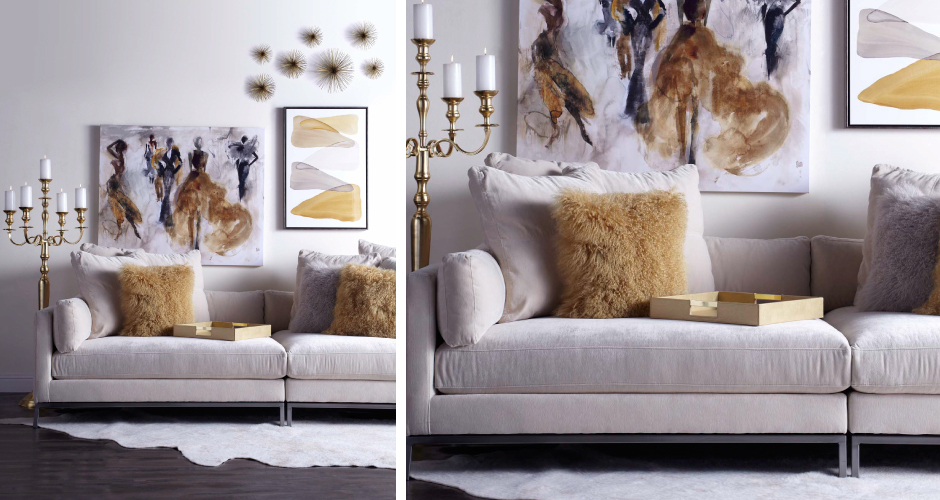
However, this is a pretty rare edge case. If you are creating your own model architecture and it simply can't fit even when you bring the batch size lower, the V100 could make sense. If you absolutely need 32 GB of memory because your model size won't fit into 11 GB of memory with a batch size of 1.If you're not sure if you need FP64, you don't. If you're doing Computational Fluid Dynamics, n-body simulation, or other work that requires high numerical precision (FP64), then you'll need to buy the Titan V or V100s. There are, however, a few key use cases where the V100s can come in handy: If you're not AWS, Azure, or Google Cloud then you're probably much better off buying the 2080 Ti.
Harmony extra deep vs zgallery ventura series#
The RTX and GTX series of cards still offers the best performance per dollar. How can the 2080 Ti be 80% as fast as the Tesla V100, but only 1/8th of the price? The answer is simple: NVIDIA wants to segment the market so that those with high willingness to pay (hyper scalers) only buy their TESLA line of cards which retail for ~$9,800. 2080 Ti vs V100 - is the 2080 Ti really that fast? Under this evaluation metric, the RTX 2080 Ti wins our contest for best GPU for Deep Learning training. We then averaged the GPU's speedup over the 1080 Ti across all models:įP32 and FP16 average speedup vs 1080 ti.įinally, we divided each GPU's average speedup by the total system cost to calculate our winner:įP32 and FP16 performance per .Unitsarespeedup/k.Unitsarespeedup/k. Throughput of each GPU on various models raw data can be found here. Speedup is a measure of the relative performance of two systems processing the same job. We divided the GPU's throughput on each model by the 1080 Ti's throughput on the same model this normalized the data and provided the GPU's per-model speedup over the 1080 Ti.
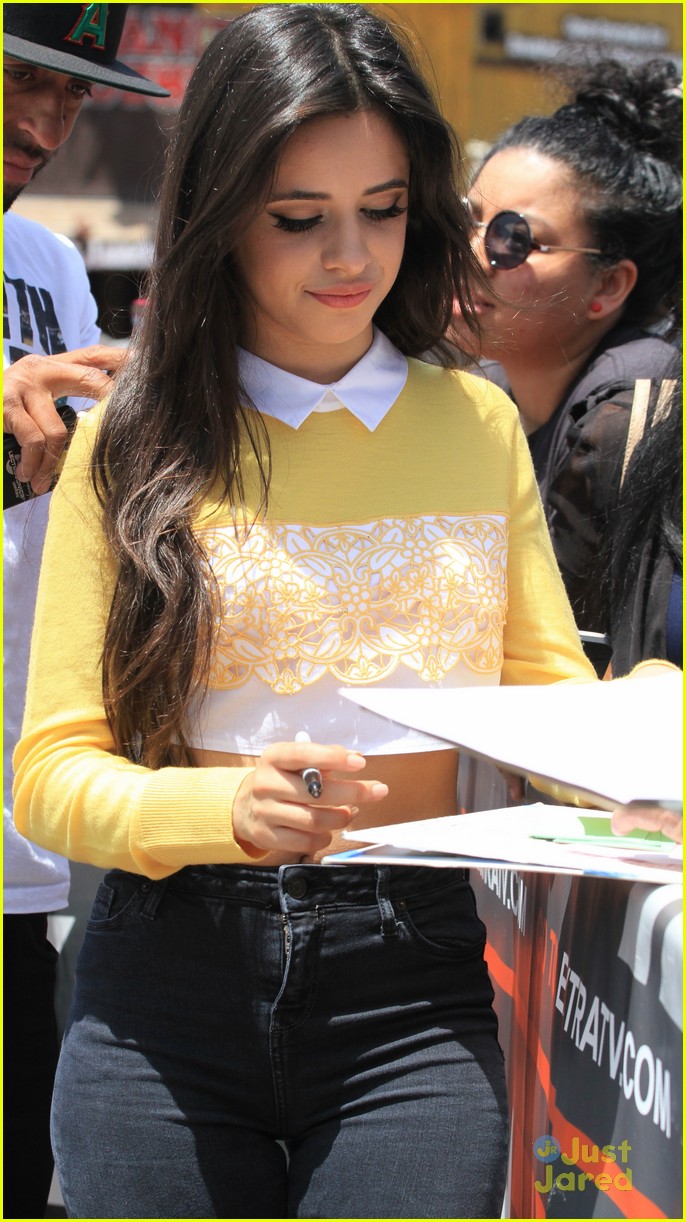
Performance of each GPU was evaluated by measuring FP32 and FP16 throughput (# of training samples processed per second) while training common models on synthetic data. You can view the benchmark data spreadsheet here. So, we've decided to make the spreadsheet that generated our graphs and (performance / $) tables public. As a system builder and AI research company, we're trying to make benchmarks that are scientific, reproducible, correlate with real world training scenarios, and have accurate prices. Note that all experiments utilized Tensor Cores when available and are priced out on a complete single GPU system cost. 80% as fast as the Tesla V100 with FP32, 82% as fast with FP16, and ~1/5 of the cost.96% as fast as the Titan V with FP32, 3% faster with FP16, and ~1/2 of the cost.35% faster than the 2080 with FP32, 47% faster with FP16, and 25% more expensive.37% faster than the 1080 Ti with FP32, 62% faster with FP16, and 25% more expensive.A typical single GPU system with this GPU will be: Results summaryĪs of February 8, 2019, the NVIDIA RTX 2080 Ti is the best GPU for deep learning research on a single GPU system running TensorFlow. To determine the best machine learning GPU, we factor in both cost and performance. At Lambda, we're often asked "what's the best GPU for deep learning?" In this post and accompanying white paper, we explore this question by evaluating the top 5 GPUs used by AI researchers:
